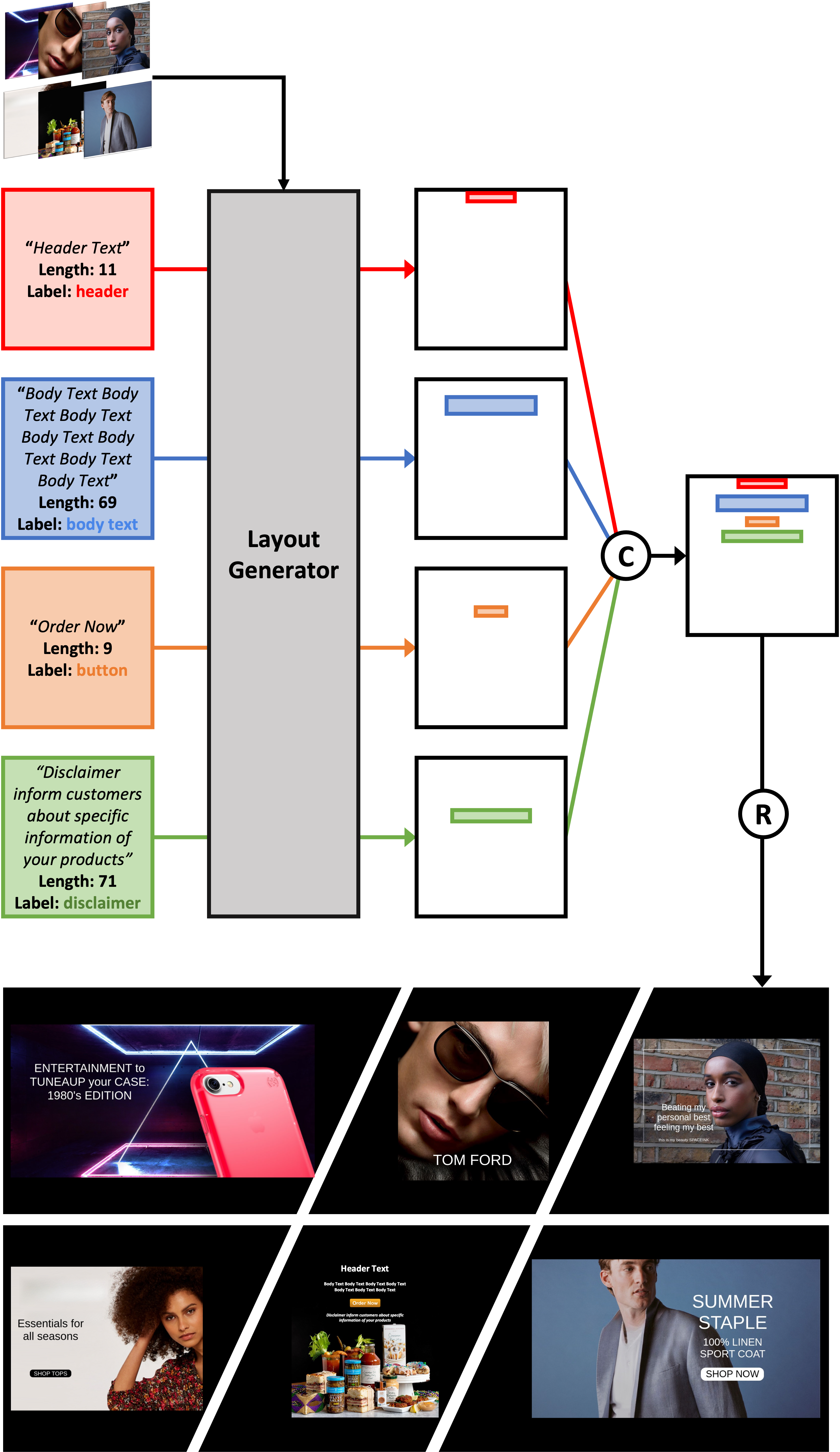
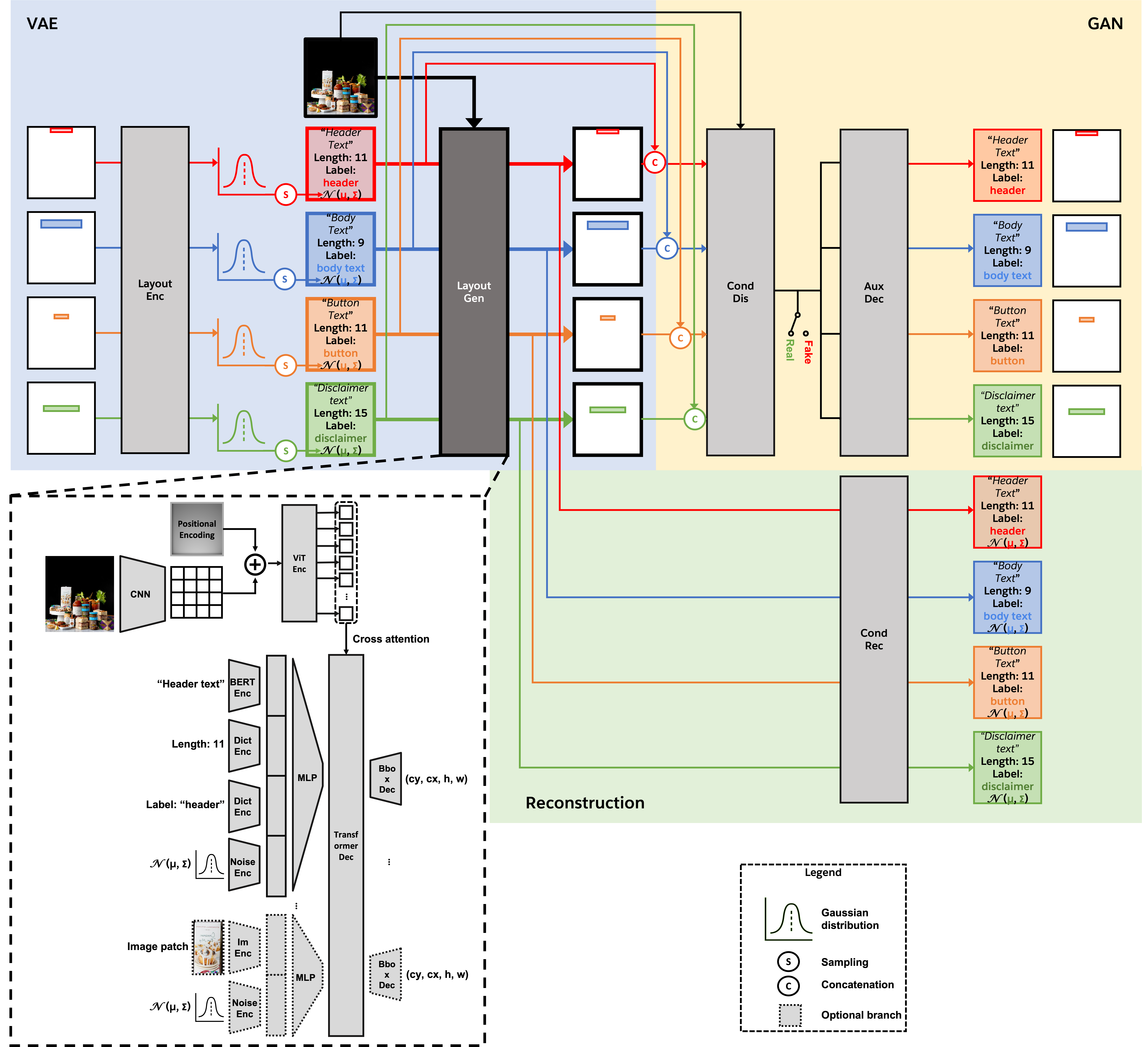
Abstract
Graphic layout designs play an essential role in visual communication. Yet handcrafting layout designs is skill-demanding, time-consuming, and non-scalable to batch production. Generative models emerge to make design automation scalable but it remains non-trivial to produce designs that comply with designers' multimodal desires, i.e., constrained by background images and driven by foreground content. We propose LayoutDETR that inherits the high quality and realism from generative modeling, while reformulating content-aware requirements as a detection problem: we learn to detect in a background image the reasonable locations, scales, and spatial relations for multimodal foreground elements in a layout. Our solution sets a new state-of-the-art performance for layout generation on public benchmarks and on our newly-curated ad banner dataset. We integrate our solution into a graphical system that facilitates user studies, and show that users prefer our designs over baselines by significant margins.
Results
Qualitative results
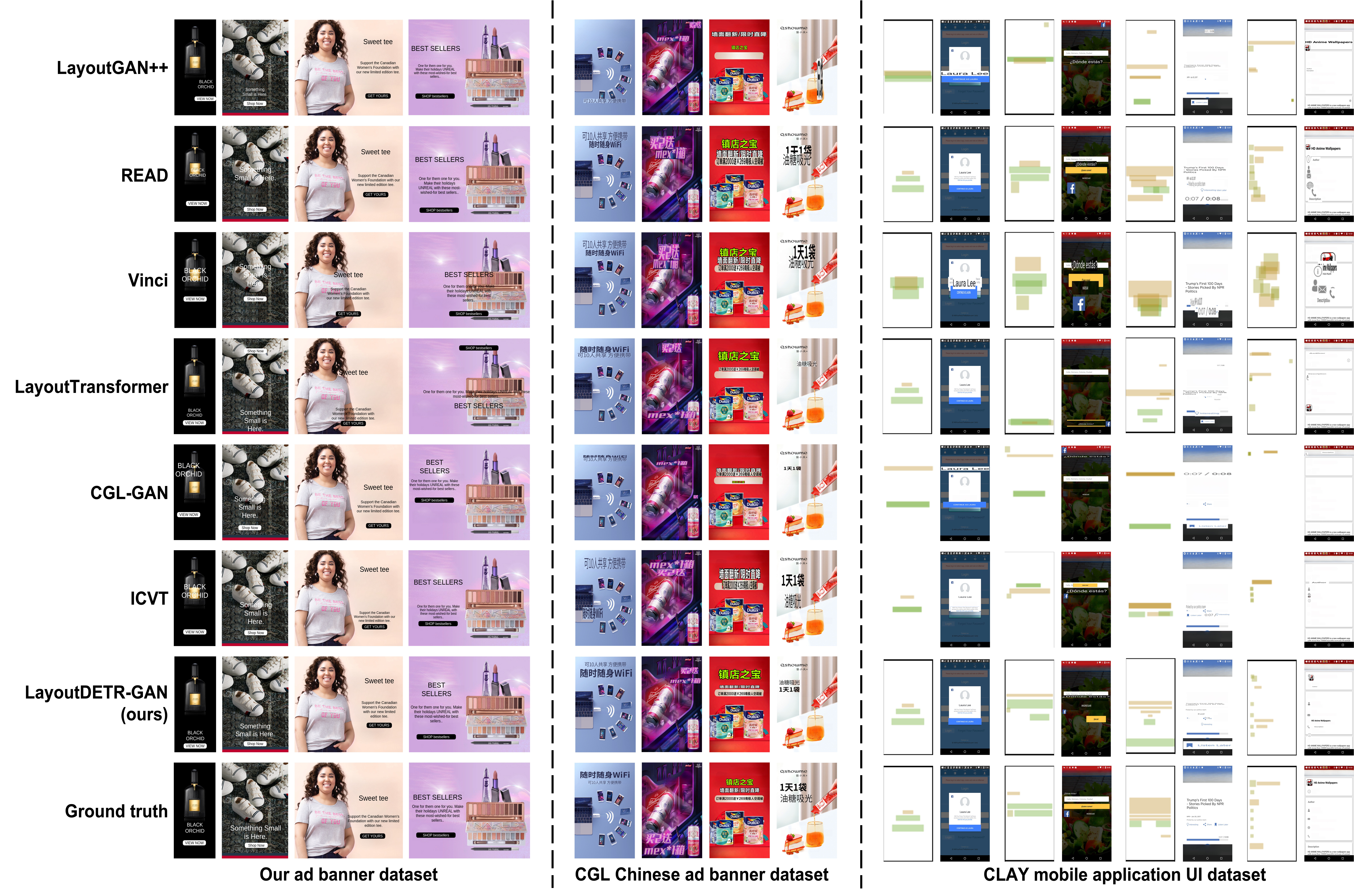
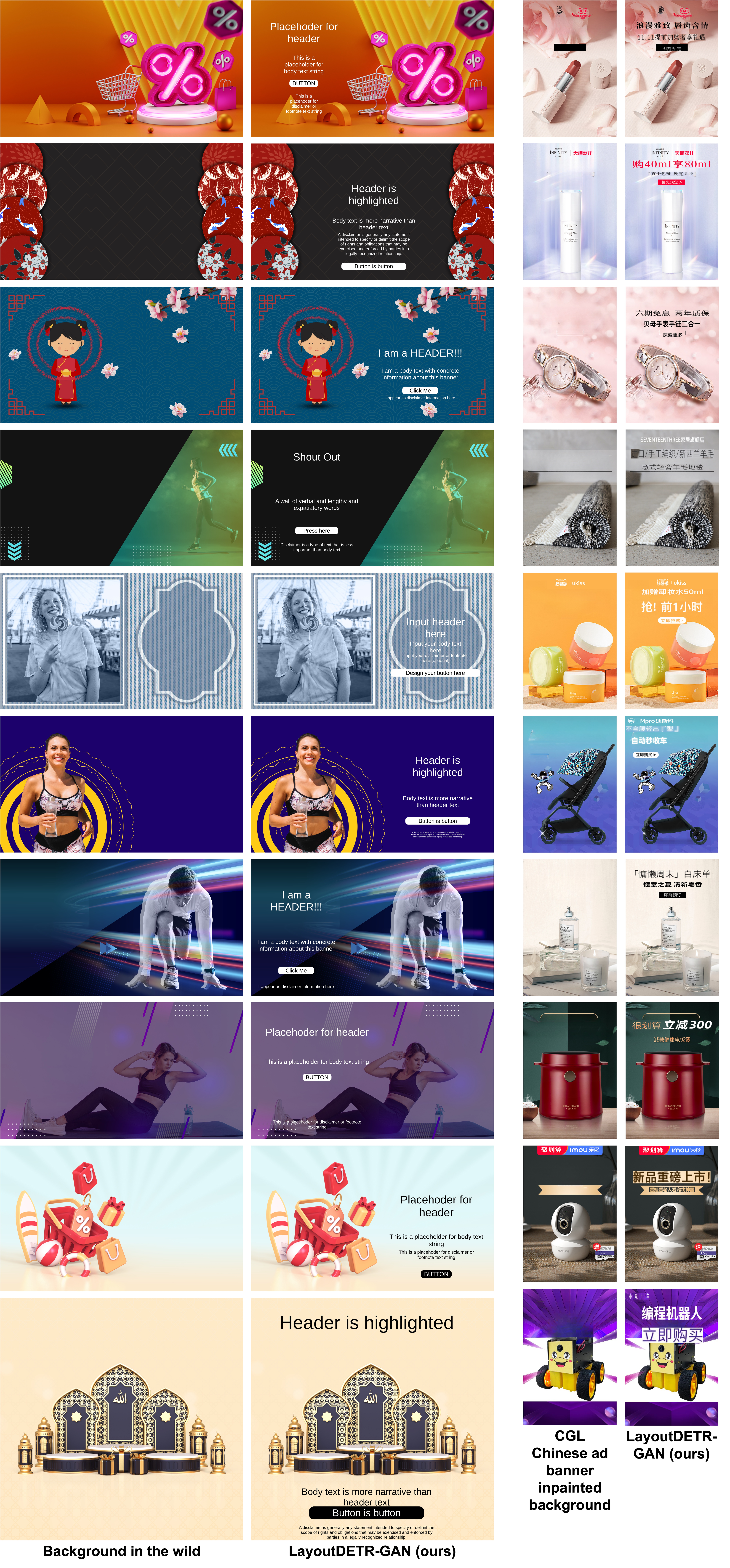
Quantitative results
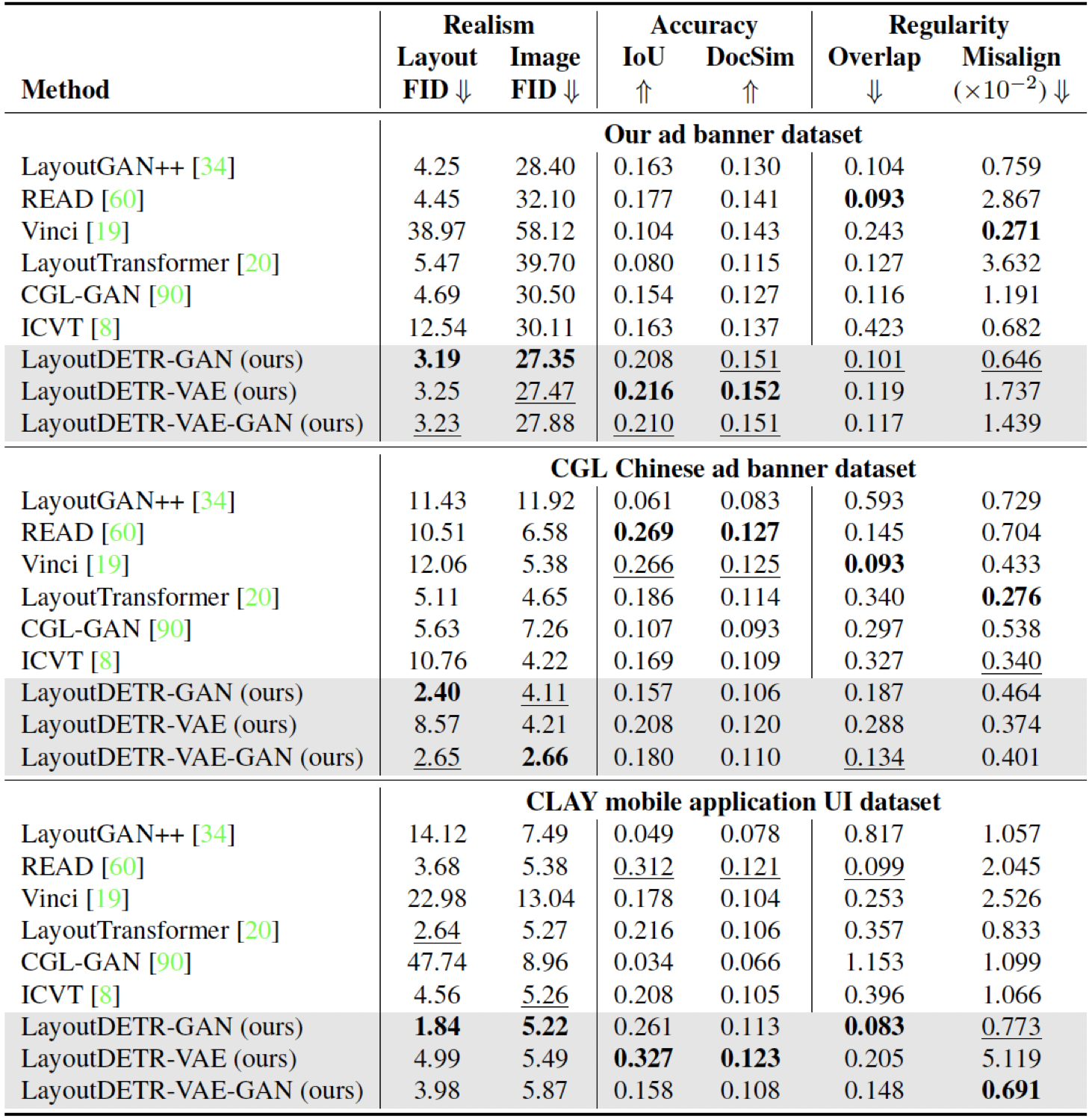
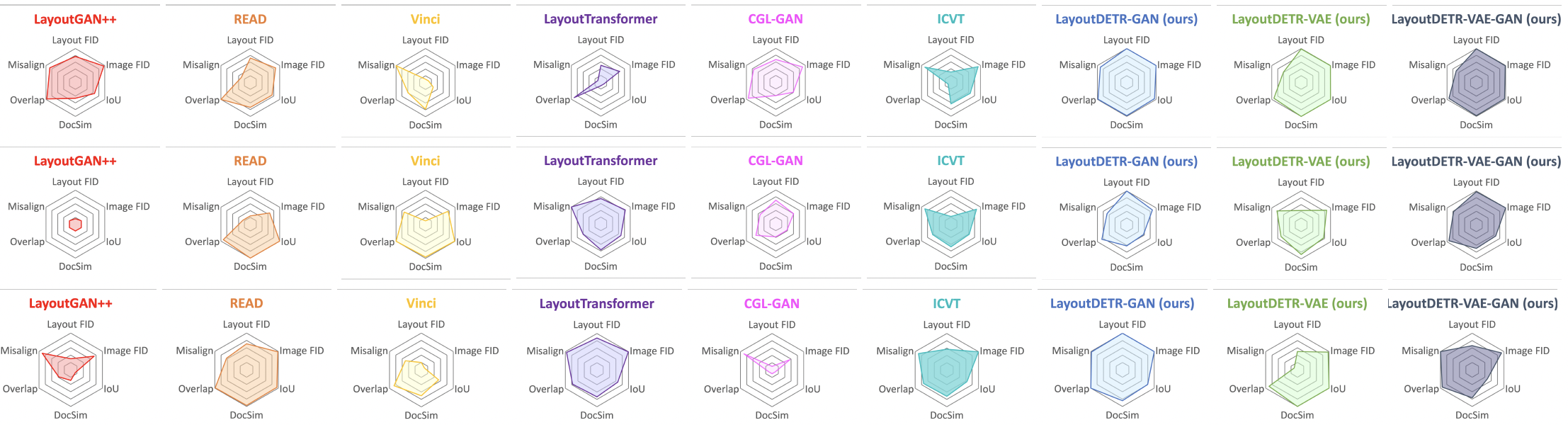
Video
Dataset
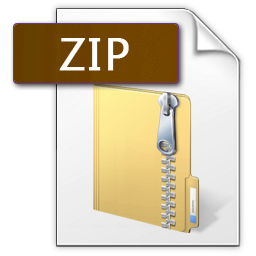
Ad banner dataset
Citation
@inproceedings{yu2024layoutdetr, title={LayoutDETR: Detection Transformer Is a Good Multimodal Layout Designer}, author={Yu, Ning and Chen, Chia-Chih and Chen, Zeyuan and Meng, Rui and Wu, Gang and Josel, Paul and Niebles, Juan Carlos and Xiong, Caiming and Xu, Ran}, booktitle={European Conference on Computer Vision (ECCV)}, year={2024} }
Acknowledgement
We thank Shu Zhang, Silvio Savarese, Abigail Kutruff, Brian Brechbuhl, Elham Etemad, and Amrutha Krishnan from Salesforce for constructive advice.