A Superpixel-Based Framework for Automatic Tumor Segmentation on Breast DCE-MRI
SPIE Medical Imaging 2015 (oral, best student paper finalist)
University of Pennsylvania
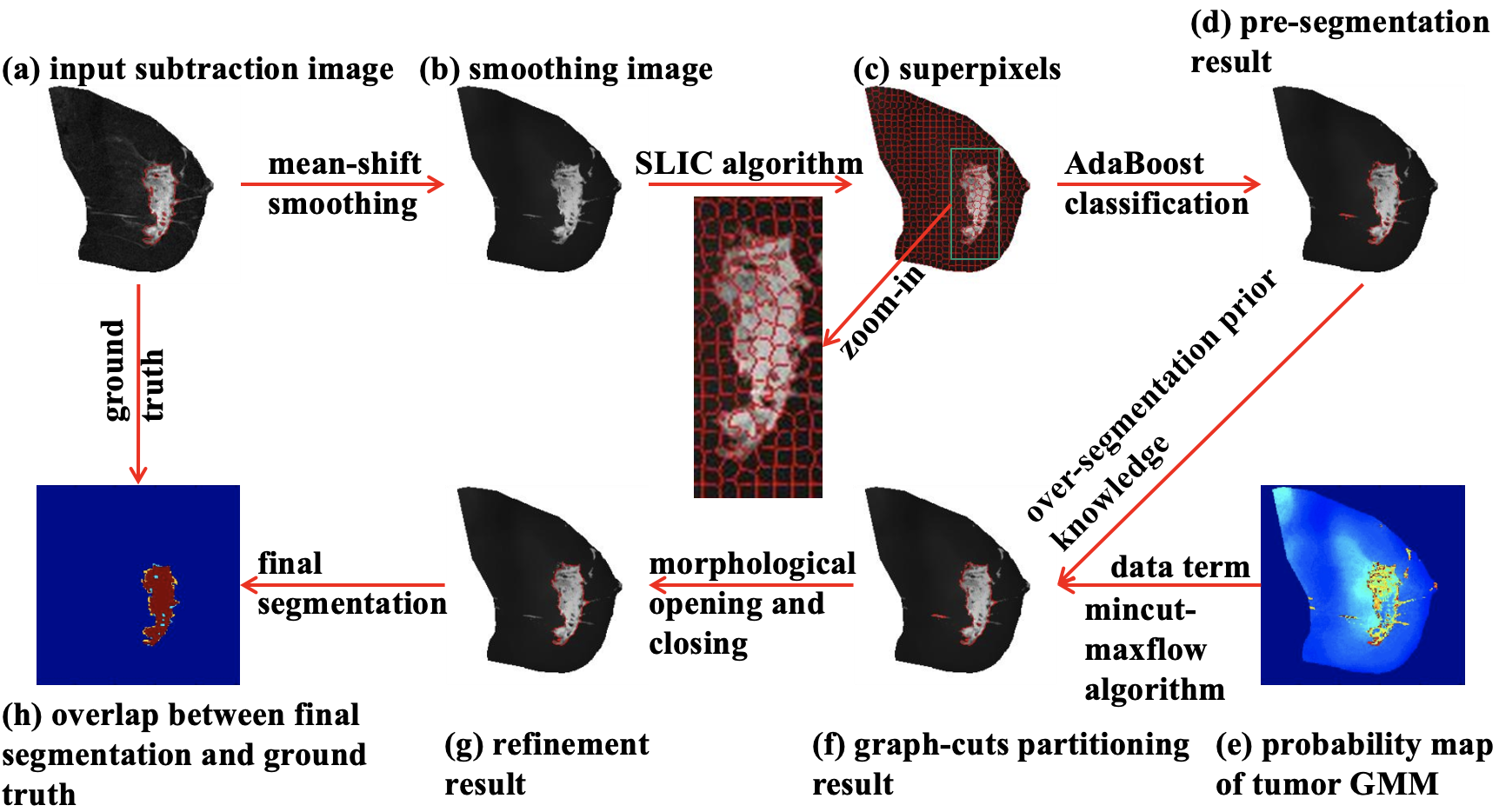
Abstract
Accurate and efficient automated tumor segmentation in breast dynamic contrast-enhanced magnetic resonance imaging (DCE-MRI) is highly desirable for computer-aided tumor diagnosis. We propose a novel automatic segmentation framework which incorporates mean-shift smoothing, superpixel-wise classification, pixel-wise graph-cuts partitioning, and morphological refinement. A set of 15 breast DCE-MR images, obtained from the American College of Radiology Imaging Network (ACRIN) 6657 I-SPY trial, were manually segmented to generate tumor masks (as ground truth) and breast masks (as regions of interest). Four state-of-the-art segmentation approaches based on diverse models were also utilized for comparison. Based on five standard evaluation metrics for segmentation, the proposed framework consistently outperformed all other approaches. The performance of the proposed framework was: 1) 0.83 for Dice similarity coefficient, 2) 0.96 for pixel-wise accuracy, 3) 0.72 for VOC score, 4) 0.79 mm for mean absolute difference, and 5) 11.71 mm for maximum Hausdorff distance, which surpassed the second best method (i.e., adaptive geodesic transformation), a semi-automatic algorithm depending on precise initialization. Our results suggest promising potential applications of our segmentation framework in assisting analysis of breast carcinomas.
Demos
Breast tumor segmentation
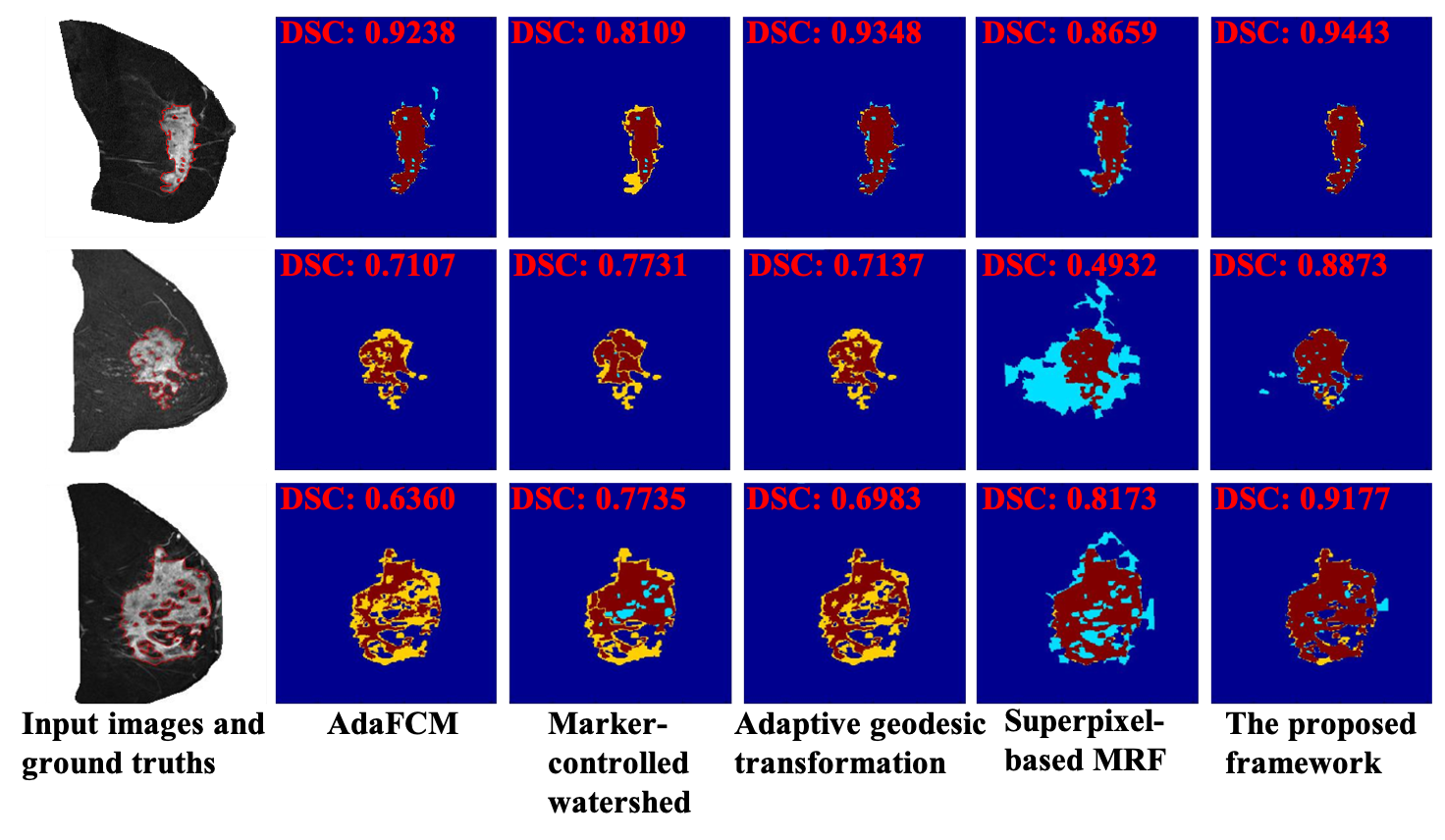
Accuracy and comparisons
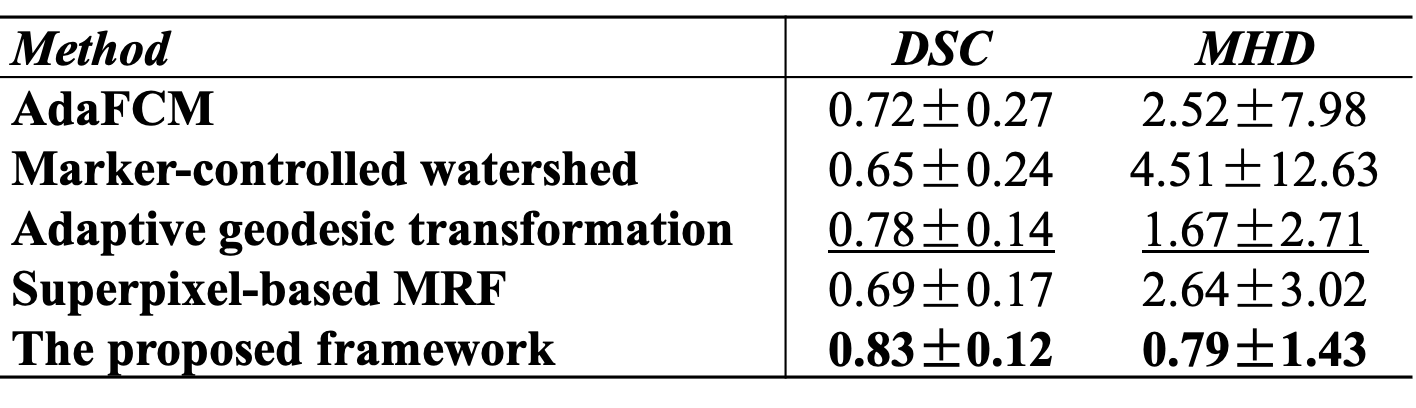
Press coverage

SPIE News
Citation
@inproceedings{yu2015superpixel, author = {Yu, Ning and Wu, Jia and Weinstein, Susan and Gaonkar, Bilwaj and Keller, Brad and Ashraf, Ahmed and Jiang, YunQing and Davatzikos, Christos and Conant, Emily and Kontos, Despina}, title = {A Superpixel-Based Framework for Automatic Tumor Segmentation on Breast DCE-MRI}, booktitle = {SPIE Medical Imaging Conference}, year = {2015} }
Acknowledgement
This project is supported by funding from the University of Pennsylvania Abramson Cancer Center 2-PREVENT Center of Excellence Program and the Center for Biomedical Image Computing and Analytics (CBICA). We thank Dr. Nola Hylton from UCSF for the permission to use the I-SPY data and the useful discussions on this research work.